Matter LLMs, Why?
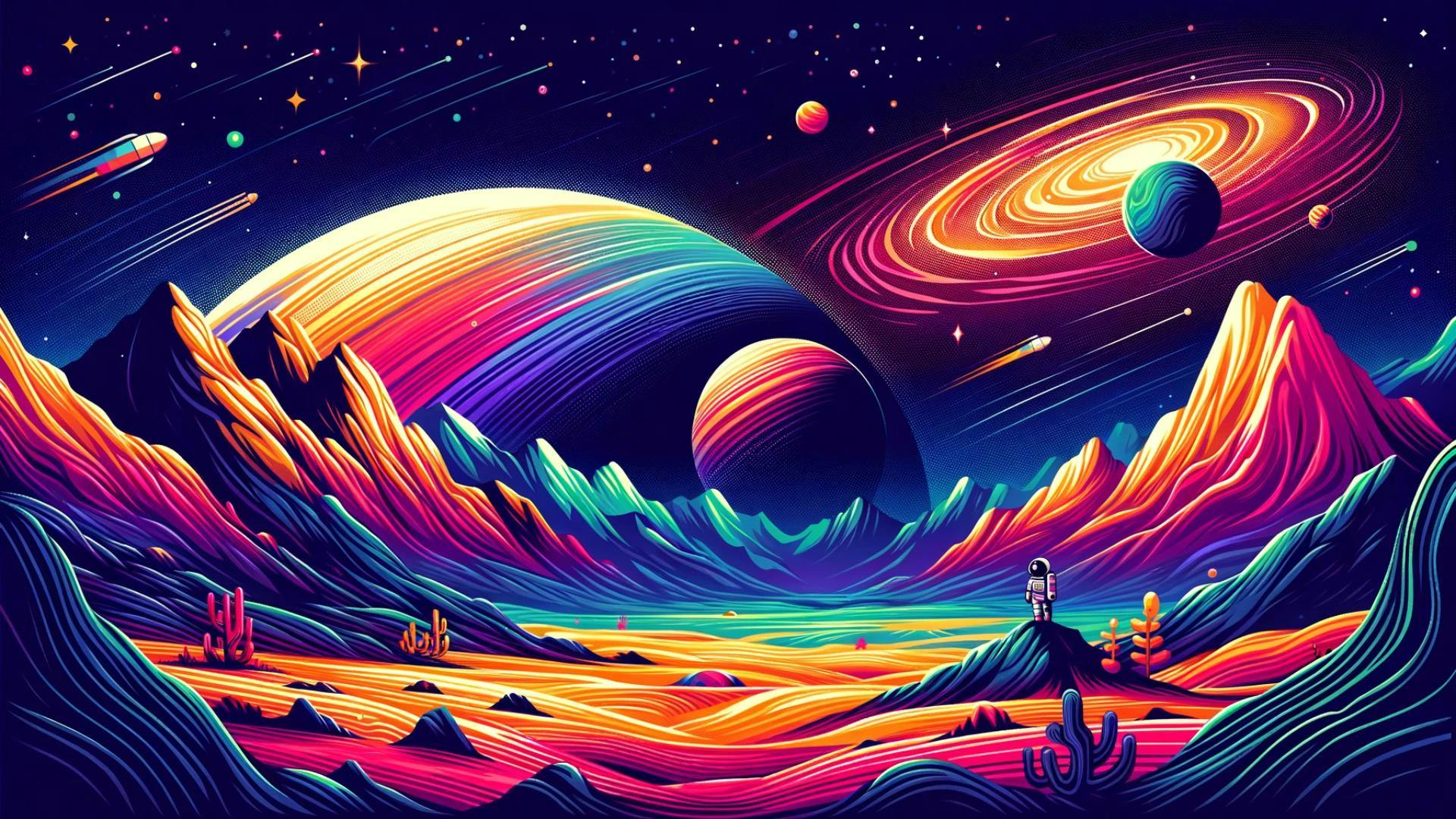
With apologies to Yoda … Matter LLMs, Why?
At the Enterprise Search & Discovery 23 conference, several speakers (Sid Probstein, Jim Ford, and Amr Awadallah) talked about neural search’s power to handle tricky keyword search cases. “Who is The Who?” for example. Another example is phrases that mean something very different when reversed. There are many great consumer examples, but there is one excellent business example: `acquire.`

If you know the companies’ names, Google will probably provide great results, but trying to find the acquisitions made by a company that may have also been acquired requires a lot of reading and query refinement.
The query `red hat acquires` is a great example. All the top results are about IBM’s massive acquisition of Red Hat; the third one, below a variety of exciting advertisements, is correct.
Keyword search depends on indexing. Like those found at the back of books, they relate pages to words. But even a sophisticated index may not retain details like the exact order of the words. (And worse, an advertising-oriented index may assume that anyone searching for anything related to Red Hat is looking for information about the company and will find what they are looking for in the first few links anyway!)
Large Language Models (LLMs) are vastly more capable interpreters of information than indexes. They’re much denser, meaning they understand, at minimum, that the order of the words is frequently all important.
Here’s Swirl’s re-ranking of Google’s top results:

The first result is correct. Google does much better if the search terms are provided as a phrase. But Google is one of the best search engines out there.
In the enterprise and within applications, it may be a different story.